Healthcare Data Analytics
Healthcare data analytics is the study of data relating to patients or services provided by a healthcare organisation. Healthcare data is extremely important to providing the very best care, but the insights available through data interpretation require expertise to extract them.
This expertise in healthcare data and analytics could be from a clinician, or it could be from a software solution, but either way there is an element of investment needed; whether that’s time, money, or belief. Without this, there will be no growth of healthcare data, but with it we can explore patterns, trends, patient habits, and make discoveries about major or minor tweaks to care processes that could save money, time, or better still save lives.
Data-driven healthcare is quickly becoming the guiding force behind modern health and care services, so in this article we will explore the importance of data in healthcare, as well provide an introduction to healthcare data analytics and explore what the analysis of healthcare data can achieve. Our goal is to make this a healthcare data guide that you can refer back to as a primer for exploring data analytics or even establishing your own analytics solutions.
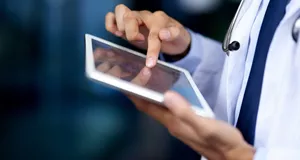
Data in Healthcare
Data in healthcare refers to information gathered about patients and the services supporting them. To make healthcare data analytics work for you though, you first need to understand why data is important in healthcare.
Why is healthcare data so valuable?
The value of healthcare data comes from the insights it can generate into a patient’s condition and the potential to catch unseen patterns, trends, or indicators that could help with treatment.
By using data in healthcare, providers can:
- Speed up diagnosis
- Identify risk factors in peoples lives
- Identify pathways of disease transmission
- Improve treatment quality and safety
- Identify aspects of healthcare education needing further engagement
This is why data-driven decision making in healthcare is becoming much more prevalent; analytics based on information we are already collecting can be utilised to work for us. This makes healthcare more proactive and switches the emphasis from reactionary to preventative, saving money, time, and most importantly protecting people from unnecessary illness or deterioration of a condition.
Types of healthcare data
There are two types of healthcare data that can be collected: qualitative data and quantitative data.
What is qualitative data in healthcare?
Qualitative data in healthcare is information that is non-numerical aka things that are observed rather than measured.
The National Institute of Health lists things such as gender, whether or not a person smokes, and yes/no answers in a questionnaire as examples of qualitative data being captured.
What is quantitative data in healthcare?
Quantitative data in healthcare is information that is numerical aka things that can be measured. This is the more traditional data captured by healthcare organisations as part of their assessment process for patients.
Again, the National Institute of Health points out the metrics that quantitative data typically captures, including things such as age, weight, patient temperature, and the number of people suffering from a specific condition.
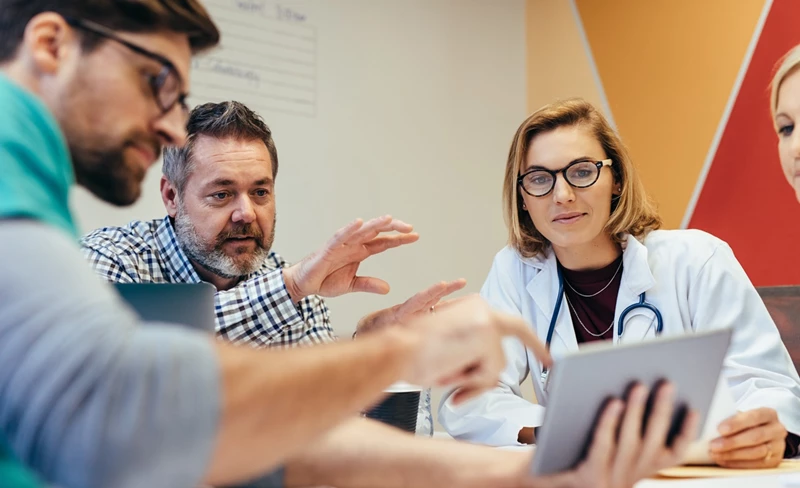
Benefits of real-time data in healthcare
The benefits of data sharing in healthcare are well known; shared information leads to improved knowledge, better actions, and typically better outcomes from more accurate treatment. In this case, real-time data is enhancing this process by being as near as possible to immediate.
This means that centralised patient records are able to be amended or updated on the spot, preventing errors from causing more mistakes than necessary, but also ensuring that patient records are as accurate as possible so that clinicians are always operating on the true patient condition and with the best possible guidance for their decision making.
The speed of real-time data is the biggest positive, and it’s from that that most of the benefits come. Quicker editing of records, easier input of data, fewer errors and they’re easier to spot at that… data precision in healthcare owes everything to real-time data.
Why is data collection important in healthcare?
The importance of data collection in healthcare boils down to the simple fact that without proper collection you can’t have proper collation, storage and observation of this intelligence. This then poses issues of time wasting in hunting down specific pieces of information and manually “connecting the dots” to link information with other information to generate the analysis required to offer crucial insights. Machine learning and automated processes can handle data collection in healthcare very quickly. Manual labour is slow and inefficient and more often than not a waste of resources.
A lack of proper data collection, perhaps due to missing or poor healthcare data architecture, can lead to false inferences or impressions. It’s also important to make use of both quantitative and qualitative data, in order to avoid bias in healthcare data or a lack of data accuracy in healthcare. For more on why is data accuracy important in healthcare you can read our blog, aptly titled ‘Why is data quality important in healthcare’.
This can have a knock-on effect of incorrect diagnosis, inappropriate treatment, and unacceptable outcomes. A good healthcare data ecosystem does not neglect the importance of data, but it also doesn’t permit unstructured data in healthcare either. Proper collation and access to clear analytics is just as important – if not more so – than the data capture in healthcare itself. Data is nothing without proper interpretation, and that can’t happen without the full picture and the tools to dissect it.
Healthcare data collection methods
Data collection tools in healthcare facilitate the gathering of information, but there are different approaches to acquiring the information, which may demand different tools. Data collection methods in healthcare typically include:
- Patient registration: NHS account, GP surgery registration, and subsequent demographics and psychographics.
- Surveys and Questionnaires
- Clinical interviews – either with a doctor or nurse
- Clinical observations
- Reviews of medical records
- Clinical trials
- Clinical treatments and the outcomes
- Biological sampling
- External data sets (socio-economic status, for example)
- Telehealth (wearables)
These are just some of the ways that healthcare data is collected, and the tools that can support this approach range from patient portal software and electronic patient records, to email management tools and survey tools to enable patient engagement.
Data analytics in healthcare
Data analytics in healthcare refers to the systematic and computational analysis of data. This is primarily in the form of statistics – quantitative data – but also accounts for the qualitative data too.
Data analytics and healthcare go together well because of the benefits generated for healthcare providers, the healthcare industry, and most importantly the patients – all of which we will touch upon soon.
Analytics, the computational analysis of data, are typically performed by healthcare software. These solutions allow the recording and cataloguing of data from different sources, and the best solutions not only allow easy access to reference the data collected and the processing of results but they also help with forecasting and predictions – much of which now ties in with AI and machine learning.
There are four types of data analytics in healthcare:
- Descriptive – Insights generated from patient history.
- Predictive – Forecasting based on the patient’s condition, history, and knowledge on an illness or condition based on existing evidence.
- Prescriptive – Proposals for patient derived from accumulated evidence. This is where the machine learning begins to have a big involvement.
- Discovery – Strategic proposals looking at bigger picture healthcare actions for regions, rather than individuals, and the trends or patterns that occur and influence wellbeing.
What is the role of data analytics in healthcare?
The role of data analytics in healthcare is to provide additional insights. In some cases this might be where clinicians and patients may not be able to spot or infer from their own experience and the available knowledge. In other cases it might refer to more overarching instances, such as the interpretation of scans or perhaps wound photographs.
The purpose, above all else, is for data to be used to its fullest potential so that patients can receive improved healthcare, which in turn should improve the care outcomes they experience.
Why is data analysis important in healthcare?
Data analysis in healthcare is important because, to use a metaphor, clinicians ‘can’t see the forest for the trees’. The patient information they are seeing is personal and individual. It is singular, and it’s only through exposure to patients and the regular work they do that they can establish trends or see patterns. This is fine, but it’s slow. Data analytics in the healthcare industry scale this up, and can provide the insights at a much quicker rate by reviewing larger numbers of patients.
This healthcare data analysis has the impact of then spotting trends earlier, allowing clinicians to adapt or prepare for a rise in conditions such as measles, for example. There’s also the potential benefits to be had for time and cost savings, which come from better usage of staff time and less wastage when treating people. The use of data analytics in healthcare is paramount if we’re to make sweeping improvements to the way modern healthcare is delivered.
AI and data analytics in healthcare
We’ve touched upon artificial intelligence in healthcare (AI) and machine learning already, but the growing impact of machine learning healthcare data is that insights are being gleaned that humans simply can’t handle based on our inconsistent language choices. Uniformity is a necessity in ensuring a lack of bias in analysis and consistency in comparisons of data sets.
Plus, as we’ve mentioned, unstructured data is an arduous task to investigate and allocate. In their blog titled Machine Learning in Healthcare, Foreseemed.com states that almost 80% of data is unstructured and buried in electronic health record solutions. This poses a problem for clinicians and the larger organisations – that is until software and machine learning steps in.
Computing is rapidly evolving year on year, and with it will come more and more data metrics we can monitor or measure. Software solutions can handle this and present this, but it will take AI and machine learning specifically to ensure these insights are generated quickly given the massive amounts of data that will be being accrued.
Of course, where there’s AI in healthcare there’s data privacy and ethics concerns. There is a moral and ethical responsibility for organisations to ensure that AI usage is respectful of existing standards and, in many cases laws, regarding personal data and the privacy afforded. We hear stories regularly about AI software scraping social media sites for information, so it’s not unbelievable that healthcare software might also act inappropriately, but this is why clinicians should be taking an early stand to enforce guidelines and rules before AI really takes a hold within healthcare.
Here at Access, our very own Alan Payne is one such expert involved in AI in healthcare. Alan is an Honorary Professor in Intelligent Systems at University College London, in addition to his role with us. Alan leads the companies AI guild in the UK, known as Ethical AI. The guild has a code of conduct, derived from Asimov’s Laws of Robotics, and applies guiding principles about aspects such as human rights, wellbeing, safety, and transparency – all to ensure that people are protected against inappropriate actions taken by AI and machine learning.
Now that we know about the role of data analytics, it’s time to evaluate the opportunities and challenges of data analytics in healthcare.
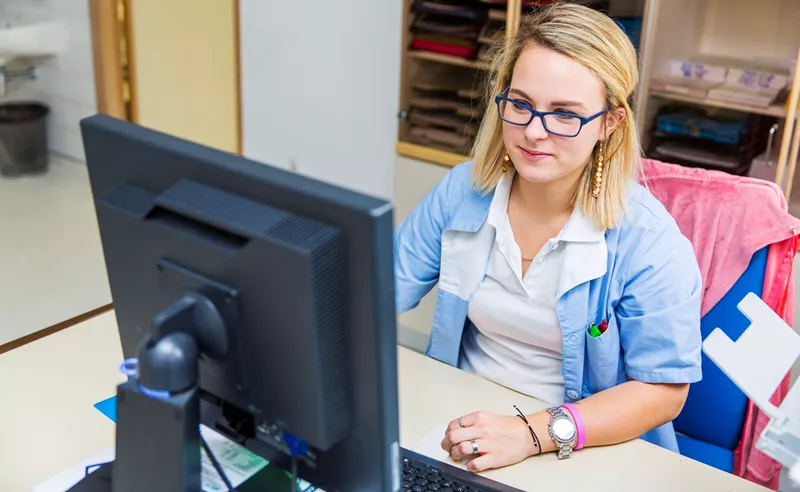
Benefits of data analytics in healthcare
Healthcare data analysis projects can improve the following for care organisations:
- Better decision making
- Real-time insights
- Informed strategic planning
- Better operational efficiency
- Optimised processes
- Better resource allocation
- Greater patient insights
- Easier patient engagement
- Greater capacity for patient activation
- Greater patient satisfaction from personalised treatment
- Better risk management
- Identify anomalies in patient data
- Spot anomalous user behaviour
- Financial opportunities
- Cost-saving opportunities
- Better budget forecasting
- Quicker adaptation to market changes
It is specifically the benefits of structured data in healthcare that achieves the above. We know the impact of data analytics in healthcare and how it can generate great insights, but the scope of the benefits is visible in just how many different aspects of healthcare it can improve. Data analytics levels up all areas of health and care.
Challenges of data analytics in healthcare
For all the benefits to be had, there are of course challenges of data analytics in healthcare, and we have touched upon some of these already.
Data protection issues in healthcare are a common topic of discussion and rightly challenged by the media, politicians, healthcare professionals and patients. The ethics of collecting and using healthcare data question to what extent people have a legal and ethical right to privacy. Whilst analytics offer a whole range of benefits, this cannot be at the expense of reasonable accommodations to maintain anonymity and to not share information beyond appropriate clinicians and services, which leads us back to our colleague and expert Alan Payne, and the Ethical AI provisions he has set out in his role as an educator and industry specialist.
So too the issue of data integrity in healthcare comes about, and the challenge of ensuring that the data gathered is factual, accurate, and timely so as not to negatively impact the analysis performed. One aspect not considered though is data interoperability in healthcare. Interoperability between software solutions, for the purpose of data sharing, is a huge ambition of organisations such as the NHS, but not all solutions offer it. Some can’t whilst other providers refuse to collaborate. This leaves organisations with an issue regarding the collation of data to ensure accurate analytics.
The final challenge comes in the form of healthcare data analysis training. Education takes time and money; both of which are finite resources in the health and care sectors. In some cases, roles might even require a PhD in healthcare data analytics due to the complexity of the computations involved. Hiring such a skilled worker costs, so it’s important that managers do their due diligence and forecast the return on investment for hiring such a specialist employee.
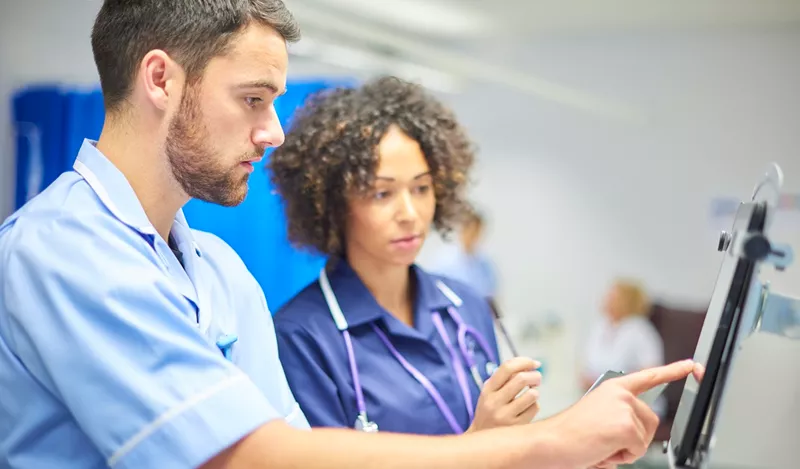
Healthcare data management
We know the opportunities and challenges of data analytics in healthcare, but we shouldn’t ignore healthcare data management, which is the handling of data to structure and collate it, thus enabling the generation of analytics.
Healthcare Data Management (HDM) takes information from electronic patient records – such as our own Rio EPR – and other solutions for things such as medication prescribing, patient flow throughout a clinical setting, and even data taken from wearables, and combines it to postulate theories based on how the data interlinks.
Healthcare data solutions
The obvious answer of how to manage data is to utilise healthcare data solutions, but the goal has to be a combination of choosing appropriate software (ideally accredited by people like the NHS or other authorities) and to ensure that these solutions can handle secure storage (ideally cloud-based), as well as role-based permissions and access tracking, to ensure legitimate and appropriate access to information. These solutions should also be capable of interoperability and securely sharing data in real-time, to get the most benefits from the intelligence.
There are bespoke healthcare data analytics companies like Arcadia and IBM who offer their services to integrate their solutions – now typically AI driven – to communicate (interoperability) with your software and collate the data available to make predictions. Some software providers, including The Access Group, have these features built into their solution, but if you’re using an existing solution that doesn’t have this capability then partnering with top healthcare data analytics companies dedicated to such analytics could be beneficial.
As is often the case with our advice, you must do your research into the healthcare data analytics market before choosing a partner organisation. Diligent investigation into the right healthcare data analytics software for you will decide whether you want to opt for a partnership with an analytics specialist, or whether you would be better served to meet multiple objectives by onboarding a new software solution that tackles a need AND offers data analytics as a by-product.
The upside is that modern healthcare software is primarily filled with data driven healthcare solutions, so there’s plenty of options to explore and opportunities to further the care your organisation provides.
Future of data analytics in healthcare
This brings us to our final talking point: the future of data analytics in healthcare. With modern technology snowballing in daily life, it won’t be too long before aspects such as AI, virtual reality, and quantum computing become very much commonplace.
Artificial intelligence and machine learning will continue to generate fresh insights as healthcare evolves and new treatments become available to the public. New illnesses might also appear though, but by being prepared with good software solutions, organisations will be ready to face the next big threat head on.
Here at Access we’re continually evaluating and evolving our health and care solutions, such as Rio Cloud – our cloud-based EPR solution. Rio Cloud offers real-time patient record management and built-in data analytics to help generate patient insights based on the available information. The real benefit is interoperability though, meaning it can share the accumulated data as well as its own findings with other partners – even specialist analytics experts.